Prepared by: Carmelo Bonannella (OpenGeoHub), Valentina Delconte (OpenGeoHub), Ichsani Wheeler (OpenGeoHub / EnvirometriX) and Tom Hengl (OpenGeoHub / EnvirometriX)
The growing interest in climate change and the potential threat it poses have seen an increasing demand for tools and solutions to the world’s greatest environmental problems. Forests are part of the solution but we have only a small window to act: forests regenerated today will have to cope with the future climate conditions of at least several decades, often even more than 100 years. How do we face this challenge? AI can help.
Tree-planting projects: more hype than solution?
Reforestation and forest restoration are considered key strategies to address climate change. Thanks to the great potential of forests in carbon sequestration, in the last two decades several reforestation programs have been conducted around the world, ranging from Latin America’s Initiative ’20 x 20’ to the Great Green Wall in sub-Saharan Africa. Europe started to sow its seeds as well: the European Union has recently committed to planting 3 billion trees in the 27 member states as a part of the European Green Deal and EU forest strategy for 2030.
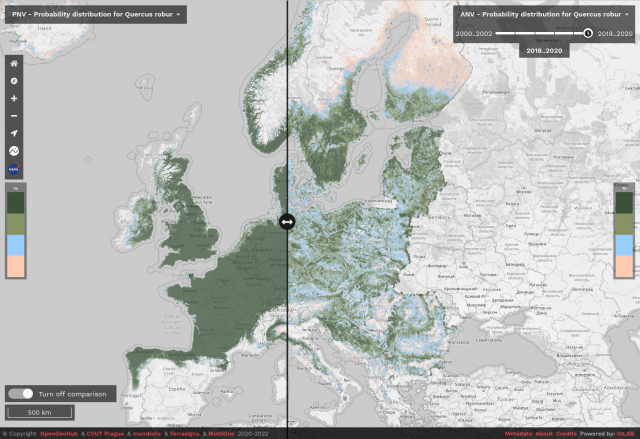
Despite great global reforestation initiatives and government pledges, planting and successfully growing trees has challenged many communities of practice, growing more concerns than actual stems. Unfortunately, ignoring or overlooking which tree grows well where has caused many tree-planting projects to fail more often than to succeed. Since its beginning in the 1980s, 80% of the multibillion-dollar 4,000-mile-long ‘Green Wall’ of planted trees in South-Saharan Africa have died; a recent study published in Nature examining reforestation efforts in Northern India, found out that decades of expensive tree planting programmes in the region have proved ineffective. These two examples of failed reforestation underpin a clear, yet overlooked pattern: in these projects, trees are often planted in sub-optimal conditions, with little to no scientific evidence about their chance of survival in the planted areas, and often without enough resources to monitor and ensure their growth over time.
“Scientists, corporations, mystics and movie stars have convinced policymakers around the world that a massive campaign to plant trees should be an essential element of global climate policy. Public dialogue has emphasized potential benefits of tree planting while downplaying pitfalls and limitations that are well established by social and ecological research.” — Forrest Fleischman, Associate Professor of Natural Resource Policy at the University of Minnesota, 2020
Vast-scale tree plantations are the most popular restoration plan, and 45% of all governmental commitments involved planting large monocultures for profit, raising serious concerns among the conservation community about their actual value in sequestering carbon and restoring resilient ecosystems. The impact of these projects can extend beyond forests’ boundaries and affect nearby ecosystems and local communities: the 2-year old Eucalyptus plantation on government-controlled land in Telangana, India, has whittled down local biodiversity, exacerbated fire risk, altered hydrology and soil condition, and restricted access to local people, disrupting community livelihoods. Even though such negative impacts are widely known across the scientific community, policymakers still struggle to recognize the damages of poorly designed tree plantings, and we are still seeking to answer the question: which tree should be planted where?
As Patrick Worms (Senior Science Policy Adviser at World Agroforestry and treasurer at the International Union for Agroforestry) puts it on LinkedIn: “Landscape restoration is never, ever just about planting trees. It’s about growing trees. The right tree, for the right purpose, in the right place. Think of it this way. Planting a tree is like having a baby. It’s not when the job stops, it’s when the job starts.”
AI for the ecosystems
In this context, OpenGeoHub has harnessed the power of AI combined with satellite data to identify the ecological requirements (temperature, precipitation, elevation etc.) of a series of keystone forest tree species (listed in the European Atlas of Forest Tree Species) covering continental Europe at 30m resolution. To give an indication of the scale, one pixel of our maps covers ⅛ of the area of a standard football pitch.
We analyzed the delicate relationship between these variables for each tree species, and translated them into potential distribution maps, showing the chance of occurrence (is the tree there?) for each tree species in each single pixel.
Just as easy as it sounds, by simply hovering the cursor over the map, it is now possible to understand if a species of interest can -or cannot- be present in that area, given its ecological requirements. While it is possible to find trees in areas where our models estimate low probability values, those areas are probably not the right place for that species and trees found there may not be healthy.
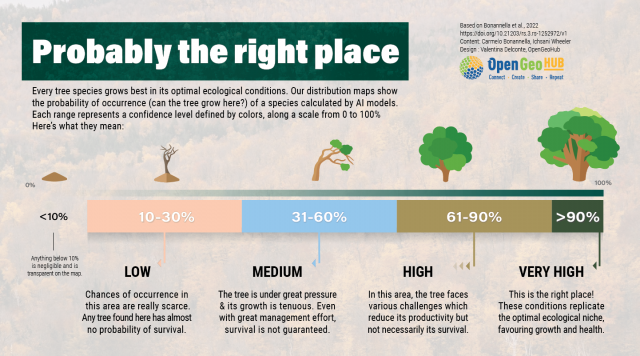
This, however, is not enough to understand what’s going on in the field: European forests have historically been subject to significant human pressures as the whole continent’s environment has been shaped by human activities. While more than ⅓ of the continent is now covered in forests, and this area is continuously growing, tree mortality in Europe is also increasing at an alarming rate due to anthropogenic and natural disturbances. Further increase in tree mortality may alter Europe’s forest demography toward younger forests, with cascading negative effects on forest natural regeneration. In a newly-formed forest patch with a certain number of tree species, young saplings compete for constantly decreasing resources. This leads more competitive species to dominate the environment, resulting in a loss of biodiversity and an impairment on ecosystem functionalities.
To get a better picture of reality, we taught our AI how different species influence each other and how human settlements and activities may impact species survival, resulting in what ecologists call realized distribution maps. These maps are significantly different from the potential distribution maps we started with; comparing them reveals the difference between the areas a species currently occupies and where it could potentially thrive.
For example, we observed both potential and realized distribution maps of the pedunculate oak across Europe (Quercus robur, L.), a continental species that plays a critical role in its ecosystem, being valued for keeping forests healthy and relevant for different insects and wildlife alike. Mankind knows this tree very well, having a long-standing relationship with it that pre-dates the Celts: this tree has provided timber for furniture, buildings and ships for centuries.
We had a look at the area currently occupied by the city of Amsterdam: note how the area could host prosperous oak forests, with the urban center being, surprisingly, one of the most suitable areas! Of course this doesn’t mean that the whole city should be replaced with trees, but it gives an idea of where in Europe a particular species could grow.
The “compare tool” available on the Open Data Science Europe viewer allows to quickly visualize the differences between potential (left) and realized (right) distribution of the pedunculate oak in the area around Amsterdam (Netherlands).
Planning 3 billion trees
Can we now give a better answer to the question “Which tree where?” — Most likely yes! Our models show that it is possible to map potentially suitable areas for different species at a level of detail useful for operational practices. These maps are not a stand-alone tool, but can already be used by experts and decision makers when planning reforestation projects.
Despite the world’s plethora of geospatial data, we’re still struggling with their real-world applications. The time is now: newly developed machine learning algorithms let us predict distribution maps with accuracy and at a cost-saving rate like never before. These types of data-driven tools can open new doors in landscape design and reforestation projects when presenting analysis- and decision-ready products. Think about it: (at least to our knowledge) no software yet exists that can be used to redesign ecosystems and building one won’t succeed without integrated designs and participation from users.
Are you interested in being an early test user for developing accessible farm-level tools? If so, contact OpenGeoHub. We are an independent not-for-profit research foundation promoting Open Source and Open Data solutions. We’re looking for landholders who are currently assessing tree-planting or agro-forestry options and are willing to provide feedback as early users with real test cases in Europe.
Do we have enough information to do this for all the species in the world? — Probably not; at least not yet. This is just an example for European species, but the potential can be expanded to virtually all known tree species in all known continents. Producing these maps was only possible thanks to countless community efforts to identify and record species occurrences in international databases such as GBIF and iNaturalist. Everyone can participate and every data point matters. So if you love nature, consider contributing to one of the citizen science projects near you!
We believe that objective, freely accessible and easy-to-use information is a valuable contribution to working out which tree goes where when planning something as substantial as planting 3 billion trees into our lands. Farmers and foresters need to lead these decisions aided by the best data that science can give them, so that we can achieve our societal goals in practical, sustainable, and profitable ways.
Accessing the forest tree species distribution data
To access the maps mentioned visit the Open Data Science Europe viewer. Maps are distributed under CC BY 4.0 license and free to download from Zenodo. Input training points, code and instructions used to prepare the maps are available here. For more information about the actual and potential predictions for forest tree species for EU please refer to:
- Bonannella, C., Hengl, T., Heisig, J., Parente, L., Wright, M.N., Herold, M., de Bruin, S., “Forest tree species distribution for Europe 2000–2020: mapping potential and realized distributions using spatiotemporal Machine Learning”, 12 January 2022, Submitted to PeerJ, PREPRINT (Version 1) available at Research Square [https://doi.org/10.21203/rs.3.rs-1252972/v1]
The OpenDataScience.eu project is co-financed by the European Union (CEF Telecom project 2018-EU-IA-0095) as a part of the Connecting Europe Facility (CEF) in Telecom programme — a key EU instrument to facilitate cross-border interaction between public administrations, businesses and citizens, by deploying digital service infrastructures (DSIs) and broadband networks.